Juni 21, 2023
EP 30 – Securing Data Amid the AI Gold Rush
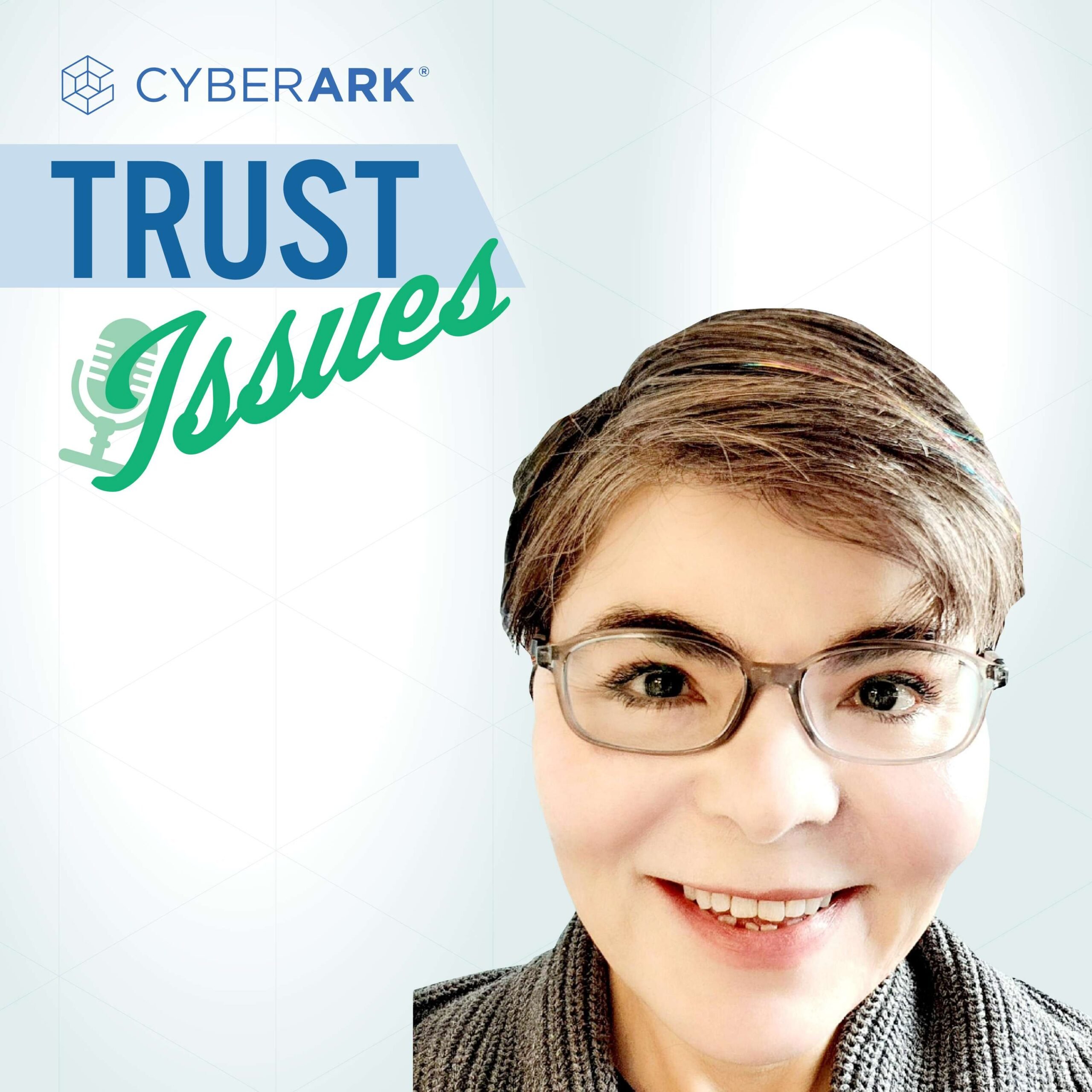
Diana Kelley, Chief Information Security Officer (CISO) at Protect AI joins host David Puner for a dive into the world of artificial intelligence (AI) and machine learning (ML), exploring the importance of privacy and security controls amid the AI Gold Rush. As the world seeks to capitalize on generative AI’s potential, risks are escalating. From protecting data from nefarious actors to addressing privacy implications and cyber threats, Kelley highlights the need for responsible AI development and usage. The conversation explores the principle of least privilege (PoLP) in AI, the privacy implications of using AI and ML platforms and the need for proper protection and controls in the development and deployment of AI and ML systems.
You’re listening to the Trust Issues podcast. I’m David Puner, a senior editorial manager at CyberArk, the global leader in Identity Security.
[00:00:08.680] – David Puner
There’s gold, and then there are AI hills. That’s right, and you well know it, the artificial intelligence gold rush is on, which means that there’s likely lots of rush development happening right now, and lots and lots of data feeding the development engine, data that needs protection so that doesn’t wind up in the wrong hands, and get used for nefarious purposes or misused or mishandled by the likes of well-intentioned developers or users of the technology. Privacy and security controls must be correctly put into place and do their thing. If they aren’t, the implications have the potential to be vast and severe.
[00:01:00.500] – David Puner
This brings us to today’s Trust Issues guest, Diana Kelley, who is the Chief Information Security Officer at Protect AI, a cybersecurity company focused on AI and ML systems. Kelley’s been thinking about AI and machine learning, since well before today’s gold rush.
[00:01:18.020] – David Puner
We take a dive into the space, and among other things are the principle of least privilege extends to AI and ML, and figures into the AI chatbot equation because data must be protected. We also talk about privacy implications, and repercussions from using AI and ML field platforms and tools, and what it all means for cyber threats and cybersecurity itself.
[00:01:42.540] – David Puner
Kelley, among lots of other highlights, is a sought after keynote speaker and co-author of two books. She’s also one of Cybersecurity Ventures‘ 100 Fascinating Females Fighting Cybercrime. She’s been driven throughout her career by the notion that technology is a wonderful tool to help humanity when it’s used right. Let’s get right into it. Here’s my talk with Diana Kelley.
[00:02:07.520] – David Puner
Diana Kelley, thank you for coming on to the Trust Issues podcast, really great to have you here.
[00:02:14.720] – Diana Kelley
Well, thank you. It’s great to be here.
[00:02:17.180] – David Puner
You’ve had a really interesting career. You’ve been with companies that include Microsoft, IBM Security and Symantec. You were CTO and co-founder of Security Curve. You serve on a number of boards, you’re a frequent keynote speaker, a conference panellist. You’re just starting week three of a new gig as the CISO at Protect AI.
[00:02:37.220] – David Puner
To start things off, before we get to today’s focus, which is artificial intelligence and machine learning, have you had a guiding principle throughout your career, and how has it led you to your latest role with Protect AI?
[00:02:50.540] – Diana Kelley
Yeah, I do. I don’t think that I realized it was a guiding principle until years later, but starting from when I fell absolutely in love with computers and connectivity, and what could be done which was in the late ’70s, I just got very excited with understanding what these systems could do, what technology could do for people to make our lives easier, and how that technology works.
[00:03:15.780] – Diana Kelley
Fast forward now, from the late ’70s when I’m a kid, and discovering all this to when I’m actually in my first couple of professional jobs in IT, and I had worked my way up to being the global manager for a Startup in Cambridge, Massachusetts. We had nine offices around the world, and we were trying to really take advantage of the technology.
[00:03:38.900] – Diana Kelley
One thing we did was, we started to issue our patches and make them available through an FTP server. I set all this up. It was great, it was wonderful. Look at what we can do. Then I realized, I hadn’t understood all the security related to setting up an FTP server, and somebody had popped it, and saw it, realized it, corrected it very quickly.
[00:03:59.460] – Diana Kelley
But at that point I was like, I need to not just focus on building these wonderful networks and systems for people to be able to use, and leverage and have benefit from. I also need to protect them from these bad guys who are trying to attack. This is the ’90s, people told me it was crazy, security wasn’t going to be essential.
[00:04:17.040] – Diana Kelley
But my guiding light of technology is a wonderful tool to help humanity, when it’s used right. Then met part of the used right is to protect it from being misused by criminals. That’s really what’s motivated me throughout my entire career.
[00:04:34.240] – Diana Kelley
As you could imagine all of that, if we take that to where we are with AI and ML, these are really powerful tools that can help humanity in so many different ways, and yet we’ve seen no end of all the different ways that they can be misused, anything from what gets a lot of attention like deepfake.
[00:04:53.660] – Diana Kelley
But there’s also things that people are not seeing such as getting misinformation from the tool and trusting it and what could be the impact of that, or even not understanding if the data that the tool is being trained on is poisoned, and what that can lead to with inaccurate or manipulated responses.
[00:05:09.980] – Diana Kelley
It’s been the same through line, it’s been different technology throughout and different focus points as security has changed, and attackers motivations have changed. But it’s always, how do we make sure that humans can get the best use out of technology?
[00:05:25.900] – David Puner
It’s always seemed to a certain degree when it comes to technology, that we’re living in the future. But now more than ever, it really does seem that we are living in the future, particularly with everything we’ve been hearing, and seeing and reading and talking about, around particularly AI but also ML in the last few months.
[00:05:43.660] – David Puner
I know you’ve really been deeply focused on it for quite some time now. As a cybersecurity professional, how are you approaching the technology and its rapid evolution and now rollout?
[00:05:55.420] – Diana Kelley
I think it always has to be, through multiple ways that we approach. There’s one which is the user, the average user of these tools. How do we make sure that they’re educated about the best way to use the tools, make sure we get the best tools into their hands, for example?
[00:06:12.040] – Diana Kelley
Then there are the people, who manage and run companies, not the ones that make the AI and ML, but maybe consuming it or doing some analytics with it. Ensuring that they as the owners, and leaders of the organizations, that they are the stewards for it, they understand how they can best use this technology, but also educate their employees on the best way to use the technology too, so that everybody’s on board.
[00:06:37.360] – Diana Kelley
Corporate environment, has to be driven by the leadership to help guide people with usage, which is a little different from commercial usage and consumer usage.
[00:06:46.260] – Diana Kelley
Then the last thing is, the creating of the AI and the ML and the developers and the builders and the engineers who are working on these systems, and ensuring that they have not only the understanding about what can go wrong so that they can build resilient systems, but also that they have the right tools to help them build those systems in the most reliable and accurate way.
[00:07:09.360] – David Puner
From an employee training standpoint, do you feel that organizations themselves understand this well enough, to be able to train their employees at this point?
[00:07:19.220] – Diana Kelley
I think there’s still a learning curve. One thing about AI and ML is that when we look at predictive analytics, intelligent analytics, a lot of what machine learning provides us, we’ve actually been in use with those for a very long time.
[00:07:33.360] – Diana Kelley
As far as educating leadership on how to use these, say you’re a financial services company, and you’ve got a Robo-advisor, you’ve got quantitative analysis and buy this and sell that. The human observes this system over time, and starts to see is this helping us? Is this not helping us? Can we tune it a little bit? Leadership, I think, does understand that it really is about accuracy and ensuring that we use them in the right way.
[00:07:59.340] – Diana Kelley
Now, as we transfer this to the big boom, which happened with ChatGPT, the large language models, and now we’ve got this whole gate opened of whether or not our search engine is going to not give us a list of things, but just give us the answer for example.
[00:08:15.800] – Diana Kelley
Or if you’re a CEO, and you’re about to acquire another company, it’s like, how do I write this email? In that case, I think that there does have to be additional understanding of what these tools can and can’t do.
[00:08:28.380] – Diana Kelley
If you ask a generative AI that’s broadly trained and that may still be getting trained on what’s available out there on the Internet, then you have to, with a grain of salt, look at the response to make sure if it’s accurate. If that sounds a little bit like an ouroboros or like a snake eating its tail, it’s because there is some element of it.
[00:08:50.280] – Diana Kelley
If you ask the system, a question that you absolutely do not know the answer to, how can you check whether that answer is accurate or not? You want that tool to be highly accurate.
[00:09:03.040] – Diana Kelley
Now, in some narrow use cases, we’re creating smaller LLMs that are very focused on a particular area. For example, when I was at IBM, we were doing this with IBM Watson for cybersecurity, and it was very honed on cybersecurity and understanding cybersecurity. We could train it, and make sure it was accurate in this fairly well-defined space.
[00:09:26.480] – Diana Kelley
A front end for a search engine that could be asked any question is probably not the best tool if you genuinely don’t know the answer to ask one of these systems that is broadly trained, that may not be highly accurate to ask it for an answer.
[00:09:42.420] – Diana Kelley
There’s the education point. We have to help leadership understand… I’m dancing around using this term, but I should use it because it’s the one that people are hearing the most, which is hallucinations. The reason that I don’t love that term, is that it makes it feel a little bit like the algorithm is sentient, and the information being responded back from that prompt is inaccurate. That’s really what’s happening.
[00:10:07.580] – David Puner
One of the other things that you mentioned, is if you’re plugging in information from an enterprise organization standpoint, for whatever it may be, an email, or you’re looking to feed some essentially data in there to figure out a way to either do something, or say something, or whatever it may be that is potentially sensitive proprietary data, that you don’t necessarily want to be plugging into these platforms. Is that safe to say?
[00:10:34.740] – Diana Kelley
It’s absolutely safe to say, that we need to understand the privacy implications and repercussions from use of these tools. We try to anonymize them, we put them into these big repositories, but very often you can reconstitute information from bits of information about somebody.
[00:10:54.680] – Diana Kelley
There’s also the privacy concern of, who’s running these models, who’s recording the information that you’re putting into the model, and can you trust them? Trusting the organizations that we’re giving data to, understanding that some of us are saying a lot more to the chatbots than we ever said to a search engine.
[00:11:12.180] – David Puner
We’ve been down this path before, with social platforms and search to much discussion, and this has been a very, very big subject for a long time. How does adding chatbots into this whole conversation when it comes to privacy, either magnify that discussion, or change that discussion? How much do we know at this point, when it comes to privacy, and chatbots and AI?
[00:11:39.560] – Diana Kelley
Especially around the LLMs and the chatbots, a lot of us are learning about what the real privacy implications in the long term are going to be. I mean, there are some real obvious ones that come out, which is when you have massive amounts of data that are available, is that data protected? Is that data anonymized properly, can it be reconstituted for an example?
[00:12:01.380] – Diana Kelley
Things that we’ve been looking at, but we have to continue and focus on in the AI space, especially now as there’s a bit of a gold rush going on. There’s this pressure to train, make these LLMs smarter, and often that means a lot more data.
[00:12:18.420] – Diana Kelley
There’s that continued view on privacy that we’ve had as we aggregate large amounts of data, but it’s just accelerated right now in the AI world, as people are just really rushing to make the best use of this technology.
[00:12:32.400] – David Puner
We’re talking about privacy in the context of what that means for cyber threats and cybersecurity itself, and all the different implications there. From a security standpoint, how should we consider both how AI and AI chatbots are built, and how they’re used, and how vulnerable they are to attacks?
[00:12:52.320] – Diana Kelley
If you’re a company who’s going to adopt it, or you’re a consumer who’s going to use it, I would look for solutions that are coming from organizations that you feel some level of trust with. I’m by no means saying that, oh, if Google made it or Microsoft made it, you’re fine, nothing bad could happen. That’s not the point.
[00:13:11.640] – Diana Kelley
The point is that these are companies that users have been interacting with, enterprises have trusted for many, many years. They do have strong security and privacy controls into place.
[00:13:23.560] – Diana Kelley
These are known quantities. I’m not saying that there’s no risk, it’s just that there’s a different risk as you look at that, than if you look at for example, using a chatbot from an organization that you’ve never heard of, that’s maybe been only in business for a couple of weeks.
[00:13:40.720] – Diana Kelley
Who knows, might be located in a nation, that has been known to be looking for data from the United States, for example.
[00:13:47.660] – David Puner
Then, how can protection and controls be built into AI and ML correctly?
[00:13:53.660] – Diana Kelley
I think one of the great things here is that we’ve got a leg up, and that we understand what a secure systems development lifecycle needs to look like. Even though AI and ML are a different development lifecycle, the transferable understanding of how to create a strong and resilient lifecycle is there.
[00:14:15.580] – Diana Kelley
You start at the very beginning with what are the requirements, what do we need this system to do? Does it need to be accurate? Does it need to have privacy and protect user information, for example?
[00:14:25.860] – Diana Kelley
Looking at AI and ML creation and deployment as part of this lifecycle, and putting the security through it, creating an architecture, and understanding the security implications of that architecture, how it’s going to be deployed, threat modelling, what’s the use, what are the misuse cases, and understanding things like failure modes.
[00:14:44.160] – Diana Kelley
That’s one of the things I did in the last couple of years was I did a class for LinkedIn Learning on failure modes that are both intentional, which is what happens when an attacker misuses, or tries to go after your AI and ML, like poisoning the data so it’s going to give inaccurate responses.
[00:15:01.020] – Diana Kelley
But there’s also unintended failure modes. The unintended ones are poor design, not doing complete testing, for example. Putting all of this into creating rigour, within your development lifecycle for AI and ML, there’s got to be a lot in between the dev and the ops to make sure it’s going to be enterprise-ready.
[00:15:21.220] – Diana Kelley
We’re looking at ML ops, and there’s a machine learning lifecycle, and then inserting the security into that. A specific case would be ML. Very often, the engineers will work within Jupyter notebooks. Within the Jupyter notebooks, they’ll have code, they’ll have data, they’ll have analytics going on in there, so that it’s really becoming in the ML space, a little bit the way that traditional developers were working inside of IDE.
[00:15:49.140] – Diana Kelley
Now you’ve got folks working inside of Jupyter notebooks. Well, what’s scanning that Jupyter notebook, if there happens to be PII in there, or if there’s a secret, like a password or an API key that’s being stored in that notebook? What’s scanning for that? We’re not scanning for that at this point.
[00:16:06.520] – Diana Kelley
Bringing the security in ML SecOps, and creating this lifecycle where we are security aware throughout the entire time of creating and deploying, and there’s a lot more there, but I’ll just plant that seed and then in the use, too.
[00:16:23.520] – Diana Kelley
That means how we use the tools that, now we’ve got, if they did ML SecOps, they should have a very robust tooling that they can deliver. Then that relates to things like if you can’t trust that this is going to be 100% accurate, don’t ask it a question that you don’t know the answer to, if you need a fully accurate answer, for example.
[00:16:43.040] – Diana Kelley
Things like, what kinds of queries, what prompts do you put into them, so that people can use these tools again, how they’re intended in the best way for the organizations and for the people who are using them.
[00:16:54.520] – David Puner
Yeah, you had some really interesting examples, in your LinkedIn learning course, like how to get an autonomous vehicle to recognize a stop sign when it’s faded. It just goes to show you all these little variables that are so consequential and anything related to this technology.
[00:17:11.660] – Diana Kelley
That’s it, and that goes to that accuracy. We do focus on ChatGPT, yet there’s accuracy there with a visual model, and the autonomous driving of… The research that I quoted, they had put some tape up onto the stop sign, but what they were trying to do was to emulate graffiti, which is very, very common.
[00:17:30.900] – Diana Kelley
The point that the researchers were trying to make, is that we see the stop sign, and it’s eight side and it’s red, and it’s in a place we expect it. Our brains just do this wonderful connect the dots.
[00:17:41.660] – Diana Kelley
Thinking back, I’ve looked at stop signs, that other than they’re in the right place and in the right shape, between how worn they’ve gotten from the sun, how many stickers and graffiti have put over it, and maybe it’s raining that day. Let’s be realistic. It really doesn’t look that much like the traditional nice bright red octagon, with stop and white big block letters.
[00:18:02.200] – Diana Kelley
As we talk about these failure modes and where they can fail, we do need to think about where we’re using AI and ML, what it’s being used for, and what could go wrong. That again, is why threat modelling is so important, and creating a systems lifecycle around AI and ML.
[00:18:18.760] – David Puner
How are AI and ML being used by threat actors to wage attacks? How is the technology itself under ongoing attack?
[00:18:27.500] – Diana Kelley
Some of the ones that have gotten a lot of noise already are using some of these generative AIs to create code. Some people were like, „Hey, create a website and it generates code, but find a zero-day exploit,“ for example. Write me a proof of concept for an attack. Writing the code that now the attackers may not even have to write it themselves. Again, they need to worry about the accuracy, but that is one way that it’s being used.
[00:18:54.520] – Diana Kelley
Another way is looking at those deepfakes I was talking about, and generating better or more believable videos.
[00:19:02.660] – Diana Kelley
Also generating and being able to do the research on somebody, to create a better phishing attack. Phishing is really dependent, on our believing that we should take this email seriously. We should do what is in the email. The way that you can get people to do things, is if you can get them to trust you, and sound authoritative.
[00:19:23.780] – Diana Kelley
Actually, if you talk to any of these chatbots, they tend to respond very confidently. Have them study, and provide an email that would be fairly likely to get somebody to click. That’s another way. There’s a little bit of bringing it into the social engineering case in these.
[00:19:41.420] – David Puner
What should organizations consider when it comes to third-party vendors and data as it applies to AI and ML?
[00:19:50.040] – Diana Kelley
If you’re looking at adopting the vendor itself, it hasn’t really caught up fully yet. But looking at one of the certification programs like SOC 2, which is very popular right now, to make sure that at least this organization is thinking about security, thinking about data protection, thinking about asset management, all of these really core baselines that should be done.
[00:20:12.740] – Diana Kelley
I think that in future, we’ll see SOC advance out a little bit more to start to really cover some of these aspects of the ML because the dev lifecycle is in there, I think it’s going to enhance to the ML lifecycle too. It’s a good certification, most companies have it. If you’re working with a company that’s got that, that’s a great start.
[00:20:32.820] – Diana Kelley
You could also ask them, if you want to go a little bit deeper, what they’ve done for their ML security lifecycle so that you can understand what they’re doing specifically around their AI and ML development.
[00:20:43.800] – Diana Kelley
Then if you’re a company that’s looking at either giving your data to a company, or using data, or even one of the pre-trained models that you can get from one of the model zoos like hugging face, is to take a deeper look at where that data comes from. What’s the provenance of it? Is it biased? Has it been cleaned properly?
[00:21:03.240] – Diana Kelley
These pre-trained models, people are adopting them because they’re great to get a really wonderful head start, but some of them have Trojan horses embedded in them, which can give attackers a view into your data that you put into the model or potentially even into seeing some other things going on within the environment that you’re training that model.
[00:21:21.940] – Diana Kelley
Being careful both to, understand any vendor that you adopt in their supply chain but expand to ML and also with the data, being very careful of what data you’re using and also what pretrained models you might be using.
[00:21:35.760] – David Puner
How does the principle of lease privilege extend into AI and ML, and how does it figure into the AI chatbot equation?
[00:21:45.180] – Diana Kelley
A big area of lease privilege around the AI and ML is the data. Who can see the data? Who can change the data? Who can see the models? Who can impact what’s going on within the lifecycle itself? You want to protect that access the way that you protect it, implement it in a different way, because now we’re in the cloud.
[00:22:05.120] – Diana Kelley
It’s similar with AI and MLs. You want to make sure that you’re not giving people access to the models, to the data, if they don’t need to have the access.
[00:22:14.780] – Diana Kelley
You also want to have good old classic separation of duties with things like training. Don’t train on production data if you don’t have to. I say that with some caveats, because as you look at what some of these use cases that are being designed, there’s going to be some blend and some crossover. But make sure that when you’re just doing the testing, who has access to that data, that they need to have access to that data.
[00:22:39.360] – Diana Kelley
As you go out and you deploy these systems in production, a lot of them, the way that you get access to the model is through an API. You can do a lot of nice lockdown business need-to-know on access to the API.
[00:22:54.120] – Diana Kelley
Does it need to be queryable by the world? Maybe, but a lot of times no. We need to take all the lessons we’ve learned, about lease privilege and business need to know, and apply it in these new systems, understanding that now we’re creating, and building in different ways that we created and built in the past.
[00:23:13.460] – David Puner
We talked about predictive ML earlier. How close are we to sentient AI? As a security practitioner, does it excite you? Do you have concerns about it?
[00:23:26.100] – Diana Kelley
Truly, sentient AI is an interesting question because we as human beings, I don’t think we understand our own intelligence entirely. I give you an example of that, if you go back to the 1940s and ’50s, in that Isaac Asimov time, there was this belief, that we were going to have robots, that were going to do pretty much all the chores that human beings don’t like to do.
[00:23:50.960] – Diana Kelley
I don’t know of anybody that’s got Rosie the Robot taped out there who can’t do laundry. This ended up being fairly complicated, and we just don’t have any solution like that, and I don’t see anything that’s coming on the horizon for that.
[00:24:05.120] – Diana Kelley
Now, what’s part of the issue there? The issue is that some of the things that we take for granted, are actually really, really hard. I use this example a lot, but picking up a glass, for example. That’s a lot of complex calculation that needs to go on. There’s some real math involved, with grip strength, with force of the muscle movement. It’s a whole lot of stuff. Human beings, we do this all the time. Well, it turns out when we try and get machines to do something like that, we have to now go deep down into all of the different complexities that are related to an action like that.
[00:24:40.420] – Diana Kelley
That’s what I mean about sentience, is that we don’t necessarily know all the intelligence even that we have. As we’re trying to create sentience, we’re going to create true intelligence. I think we’re still learning a lot about what intelligence actually means. What else do we need to get into those systems to make them truly sentient?
[00:25:03.380] – Diana Kelley
There’s almost a metaphysical, philosophical conversation too. What does it mean to have a mind? There are a couple of very, very deep AI researchers, who have started to be concerned about this, but most of the scientists in the field are not concerned about the general sentient AI. It’s really more about making sure that the AI that we have available now, the narrower AI is used in a responsible, reliable, and ethical way.
[00:25:32.740] – Diana Kelley
I do think there’s a lot more steps towards true sentience, and a lot more about intelligence that we don’t understand for ourselves. I think that’s the intersection point we are at right now. Societally, it’s, „Oh my gosh, ChatGPT can pass the bar, and I know people that are so smart that couldn’t do it.“ But passing the bar is not the same as having general sentience and intelligence.
[00:25:56.320] – David Puner
A bunch of really interesting points there. I appreciate that. If you were to beam yourself back to when you were just starting your career, what’s most surprising about your career journey and the field itself?
[00:26:09.260] – Diana Kelley
I think most surprising is that I was actually able to succeed in it because I didn’t think that technology was going to be a career for me. Anybody who thinks maybe you don’t belong in tech, remember that, that not everybody who has succeeded in tech thought that we belonged here at the beginning. I just want to encourage people to support yourself, because that’s a really important thing.
[00:26:32.580] – Diana Kelley
But the biggest surprise within the industry, since I got started is just how pervasive and everywhere it is. I got excited about technology in the late ’70s. I thought it was the future, but I didn’t realize how it was going to be literally everywhere. I never thought it would be something that was going to be at the level of defending nations, and ensuring that they can communicate and defend themselves at times of war. I mean, that’s pretty intense. Or even making sure that we can be safe as we’re driving our cars.
[00:27:02.180] – David Puner
Diana Kelley, thanks so much.
[00:27:03.820] – Diana Kelley
Thank you. It’s great to be here.
[00:27:06.140] – David Puner
Thanks for listening to Trust Issues. If you like this episode, please check out our back catalogue for more conversations with cyber defenders and protectors. Don’t miss new episodes. Make sure you’re following us wherever you get your podcasts.
[00:27:30.040] – David Puner
Let’s see, drop us a line if you feel so inclined, questions, comments, suggestions, which come to think of it, are comments. Our email address is [email protected]. See you next time.